Optimization selection and incentives of client in online asynchronous federated learning
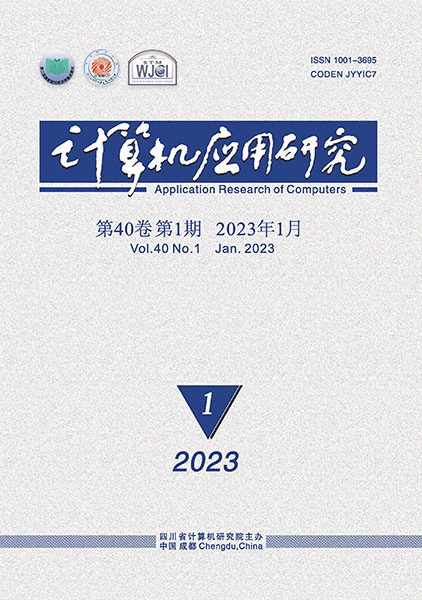
Abstract
Key Words
Foundation Support
Publish Information
Publish History
Cite This Article
About the Journal
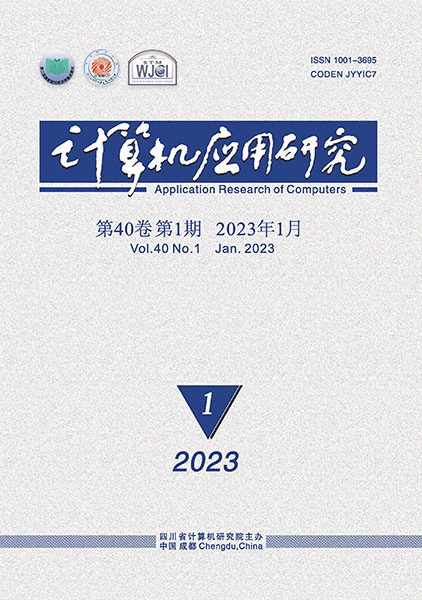
- Application Research of Computers Monthly Journal
-
Journal ID
ISSN 1001-3695
CN 51-1196/TP
Application Research of Computers, founded in 1984, is an academic journal of computing technology sponsored by Sichuan Institute of Computer Sciences under the Science and Technology Department of Sichuan Province.
Aiming at the urgently needed cutting-edge technology in this discipline, Application Research of Computers reflects the mainstream technology, hot technology and the latest development trend of computer application research at home and abroad in a timely manner. The main contents of the journal include high-level academic papers in this discipline, the latest scientific research results and major application results. The contents of the columns involve new theories of computer discipline, basic computer theory, algorithm theory research, algorithm design and analysis, blockchain technology, system software and software engineering technology, pattern recognition and artificial intelligence, architecture, advanced computing, parallel processing, database technology, computer network and communication technology, information security technology, computer image graphics and its latest hot application technology.
Application Research of Computers has many high-level readers and authors, and its readers are mainly senior and middle-level researchers and engineers engaged in the field of computer science, as well as teachers and students majoring in computer science and related majors in colleges and universities. Over the years, the total citation frequency and Web download rate of Application Research of Computers have been ranked among the top of similar academic journals in this discipline, and the academic papers published are highly popular among the readers for their novelty, academics, foresight, orientation and practicality.
Indexed & Evaluation
- The Second National Periodical Award 100 Key Journals
- Double Effect Journal of China Journal Formation
- the Core Journal of China (Peking University 2023 Edition)
- the Core Journal for Science
- Chinese Science Citation Database (CSCD) Source Journals
- RCCSE Chinese Core Academic Journals
- Journal of China Computer Federation
- 2020-2022 The World Journal Clout Index (WJCI) Report of Scientific and Technological Periodicals
- Full-text Source Journal of China Science and Technology Periodicals Database
- Source Journal of China Academic Journals Comprehensive Evaluation Database
- Source Journals of China Academic Journals (CD-ROM Version), China Journal Network
- 2017-2019 China Outstanding Academic Journals with International Influence (Natural Science and Engineering Technology)
- Source Journal of Top Academic Papers (F5000) Program of China's Excellent Science and Technology Journals
- Source Journal of China Engineering Technology Electronic Information Network and Electronic Technology Literature Database
- Source Journal of British Science Digest (INSPEC)
- Japan Science and Technology Agency (JST) Source Journal
- Russian Journal of Abstracts (AJ, VINITI) Source Journals
- Full-text Journal of EBSCO, USA
- Cambridge Scientific Abstracts (Natural Sciences) (CSA(NS)) core journals
- Poland Copernicus Index (IC)
- Ulrichsweb (USA)