困难样本采样联合对比增强的深度图聚类
Deep graph clustering with hard sample sampling joint contrastive augmentation
云南大学 信息学院, 昆明 650504
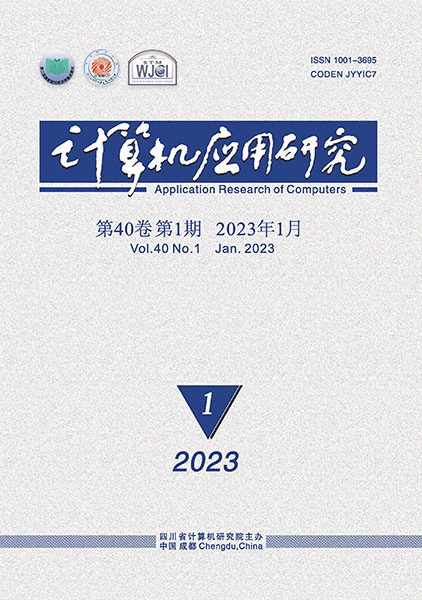
摘要
针对困难样本挖掘的图聚类算法是最近的研究热点,目前算法存在的主要问题有:对比方法和样本对加权策略缺少良好的融合机制;采样正样本时忽略了视图内部的“假阴性”样本;忽视图级信息对聚类的帮助。针对上述问题,提出困难样本采样联合对比增强的图聚类算法。首先使用自编码器学习嵌入,根据计算的伪标签、相似度、置信度信息为表示学习设计一种自加权对比损失,统一不同视图下节点对比和困难样本对加权策略。通过调整不同置信区域样本对的权重,损失函数驱动模型关注不同类型的困难样本以学习有区分性的特征,提高簇内表示的一致性和簇间表示的差异性,增强对样本的判别能力。其次,图级表示经聚类网络投影,通过聚类对比损失最大化不同视图下聚类的表示一致性。最后联合两种对比损失,利用自监督训练机制进行迭代优化,完成聚类任务。该算法在5个真实数据集上与9个基准聚类算法对比,在4个权威指标上达到最优,聚类性能出色。消融实验表明两个对比模块的有效性和可迁移性。
基金项目
国家自然科学基金资助项目(62062066,61762090,61966036,62276227)
2022年云南省基础研究计划重点项目(202201AS070015)
云南省中青年学术和技术带头人后备人才资助项目(202205AC160033)
云南省智能系统与计算重点实验室资助项目(202205AG070003)
出版信息
DOI: 10.19734/j.issn.1001-3695.2023.10.0521
出版期卷: 《计算机应用研究》 印刷出版, 2024年第41卷 第6期
所属栏目: 算法研究探讨
出版页码: 1769-1777
文章编号: 1001-3695(2024)06-024-1769-09
发布历史
[2024-01-16] 优先出版
[2024-06-05] 印刷出版
引用本文
朱玄烨, 孔兵, 陈红梅, 等. 困难样本采样联合对比增强的深度图聚类 [J]. 计算机应用研究, 2024, 41 (6): 1769-1777. (Zhu Xuanye, Kong Bing, Chen Hongmei, et al. Deep graph clustering with hard sample sampling joint contrastive augmentation [J]. Application Research of Computers, 2024, 41 (6): 1769-1777. )
关于期刊
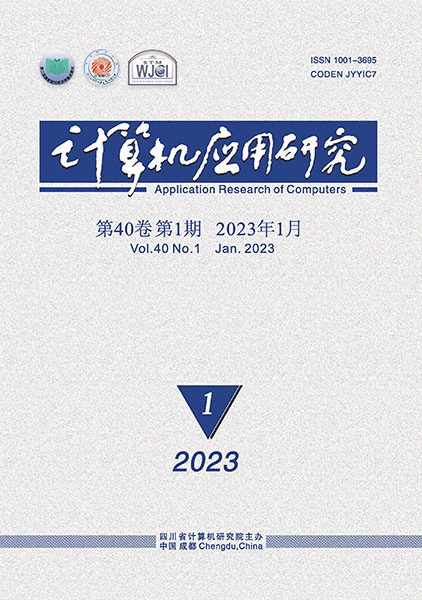
- 计算机应用研究 月刊
- Application Research of Computers
-
刊号
ISSN 1001-3695
CN 51-1196/TP
《计算机应用研究》创刊于1984年,是由四川省科技厅所属四川省计算机研究院主办的计算技术类学术刊物。
《计算机应用研究》瞄准本学科领域迫切需要的前沿技术,及时反映国内外计算机应用研究的主流技术、热点技术及最新发展趋势。主要刊载内容包括本学科领域高水平的学术论文、本学科最新科研成果和重大应用成果。栏目内容涉及计算机学科新理论、计算机基础理论、算法理论研究、算法设计与分析、区块链技术、系统软件与软件工程技术、模式识别与人工智能、体系结构、先进计算、并行处理、数据库技术、计算机网络与通信技术、信息安全技术、计算机图像图形学及其最新热点应用技术。
《计算机应用研究》拥有众多高层次读者、作者,读者对象主要为从事计算机学科领域高、中级研究人员及工程技术人员,各高等院校计算机专业及相关专业的师生。多年来《计算机应用研究》的总被引频次及Web下载率一直名列本学科同类学术刊物前茅,所刊发的学术论文以其新颖性、学术性、前瞻性、导向性、实用性而备受广大读者的喜爱。
收录和评价
- 第二届国家期刊奖百种重点期刊
- 中国期刊方阵双效期刊
- 全国中文核心期刊(北大2023年版)
- 中国科技核心期刊
- 中国科学引文数据库(CSCD)来源期刊
- RCCSE中国核心学术期刊
- 中国计算机学会会刊
- 2020—2022年科技期刊世界影响力指数(WJCI)报告收录期刊
- 中国科技期刊精品数据库全文来源期刊
- 中国学术期刊综合评价数据库来源期刊
- 《中国期刊网》《中国学术期刊(光盘版)》来源期刊
- 2017—2019年中国国际影响力优秀学术期刊(自然科学与工程技术)
- 中国精品科技期刊顶尖学术论文(F5000)项目来源期刊
- 《中国工程技术电子信息网》《电子科技文献数据库》来源期刊
- 英国《科学文摘》(INSPEC)来源期刊
- 《日本科学技术振兴机构数据库》(JST)来源期刊
- 俄罗斯《文摘杂志》(AJ, VINITI)来源期刊
- 美国《艾博思科学术数据库》(EBSCO)全文来源期刊
- 美国《剑桥科学文摘(自然科学)》(CSA(NS))核心期刊
- 波兰《哥白尼索引》(IC)来源期刊
- 美国《乌利希期刊指南(网络版)》(Ulrichsweb)收录期刊